Amazon Web Services Feed
Improving speech-to-text transcripts from Amazon Transcribe using custom vocabularies and Amazon Augmented AI
Businesses and organizations are increasingly using video and audio content for a variety of functions, such as advertising, customer service, media post-production, employee training, and education. As the volume of multimedia content generated by these activities proliferates, businesses are demanding high-quality transcripts of video and audio to organize files, enable text queries, and improve accessibility to audiences who are deaf or hard of hearing (466 million with disabling hearing loss worldwide) or language learners (1.5 billion English language learners worldwide).
Traditional speech-to-text transcription methods typically involve manual, time-consuming, and expensive human labor. Powered by machine learning (ML), Amazon Transcribe is a speech-to-text service that delivers high-quality, low-cost, and timely transcripts for business use cases and developer applications. In the case of transcribing domain-specific terminologies in fields such as legal, financial, construction, higher education, or engineering, the custom vocabularies feature can improve transcription quality. To use this feature, you create a list of domain-specific terms and reference that vocabulary file when running transcription jobs.
This post shows you how to use Amazon Augmented AI (Amazon A2I) to help generate this list of domain-specific terms by sending low-confidence predictions from Amazon Transcribe to humans for review. We measure the word error rate (WER) of transcriptions and number of correctly-transcribed terms to demonstrate how to use custom vocabularies to improve transcription of domain-specific terms in Amazon Transcribe.
To complete this use case, use the notebook A2I-Video-Transcription-with-Amazon-Transcribe.ipynb on the Amazon A2I Sample Jupyter Notebook GitHub repo.
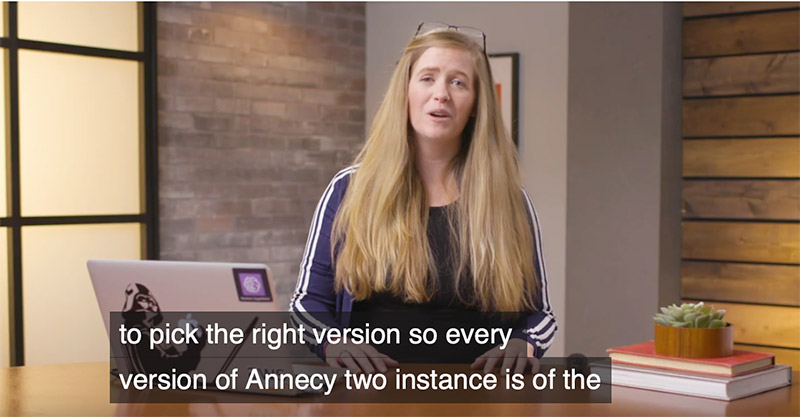
Example of mis-transcribed annotation of the technical term, “an EC2 instance”. This term was transcribed as “Annecy two instance”.
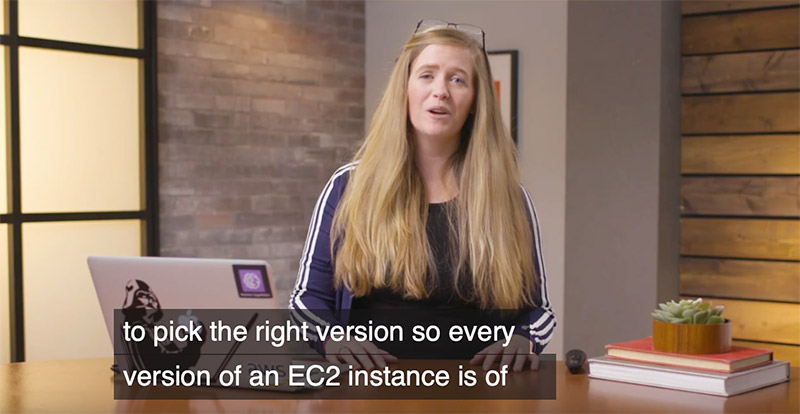
Example of correctly transcribed annotation of the technical term “an EC2 instance” after using Amazon A2I to build an Amazon Transcribe custom vocabulary and re-transcribing the video.
This walkthrough focuses on transcribing video content. You can modify the code provided to use audio files (such as MP3 files) by doing the following:
- Upload audio files to your Amazon Simple Storage Service (Amazon S3) bucket and using them in place of the video files provided.
- Modify the button text and instructions in the worker task template provided in this walkthrough and tell workers to listen to and transcribe audio clips.
Solution overview
The following diagram presents the solution architecture.
We briefly outline the steps of the workflow as follows:
- Perform initial transcription. You transcribe a video about Amazon SageMaker, which contains multiple mentions of technical ML and AWS terms. When using Amazon Transcribe out of the box, you may find that some of these technical mentions are mis-transcribed. You generate a distribution of confidence scores to see the number of terms that Amazon Transcribe has difficulty transcribing.
- Create human review workflows with Amazon A2I. After you identify words with low-confidence scores, you can send them to a human to review and transcribe using Amazon A2I. You can make yourself a worker on your own private Amazon A2I work team and send the human review task to yourself so you can preview the worker UI and tools used to review video clips.
- Build custom vocabularies using A2I results. You can parse the human-transcribed results collected from Amazon A2I to extract domain-specific terms and use these terms to create a custom vocabulary table.
- Improve transcription using custom vocabulary. After you generate a custom vocabulary, you can call Amazon Transcribe again to get improved transcription results. You evaluate and compare the before and after performances using an industry standard called word error rate (WER).
Prerequisites
Before beginning, you need the following:
- An AWS account.
- An S3 bucket. Provide its name in
BUCKET
in the notebook. The bucket must be in the same Region as this Amazon SageMaker notebook instance. - An AWS Identity and Access Management (IAM) execution role with required permissions. The notebook automatically uses the role you used to create your notebook instance (see the next item in this list). Add the following permissions to this IAM role:
- Attach managed policies
AmazonAugmentedAIFullAccess
andAmazonTranscribeFullAccess
. - When you create your role, you specify Amazon S3 permissions. You can either allow that role to access all your resources in Amazon S3, or you can specify particular buckets. Make sure that your IAM role has access to the S3 bucket that you plan to use in this use case. This bucket must be in the same Region as your notebook instance.
- Attach managed policies
- An active Amazon SageMaker notebook instance. For more information, see Create a Notebook Instance. Open your notebook instance and upload the notebook A2I-Video-Transcription-with-Amazon-Transcribe.ipynb.
- A private work team. A work team is a group of people that you select to review your documents. You can choose to create a work team from a workforce, which is made up of workers engaged through Amazon Mechanical Turk, vendor-managed workers, or your own private workers that you invite to work on your tasks. Whichever workforce type you choose, Amazon A2I takes care of sending tasks to workers. For this post, you create a work team using a private workforce and add yourself to the team to preview the Amazon A2I workflow. For instructions, see Create a Private Workforce. Record the ARN of this work team—you need it in the accompanying Jupyter notebook.
To understand this use case, the following are also recommended:
- Basic understanding of AWS services like Amazon Transcribe, its features such as custom vocabularies, and the core components and workflow Amazon A2I uses.
- The notebook uses the AWS SDK for Python (Boto3) to interact with these services.
- Familiarity with Python and NumPy.
- Basic familiarity with Amazon S3.
Getting started
After you complete the prerequisites, you’re ready to deploy this solution entirely on an Amazon SageMaker Jupyter notebook instance. Follow along in the notebook for the complete code.
To start, follow the Setup code cells to set up AWS resources and dependencies and upload the provided sample MP4 video files to your S3 bucket. For this use case, we analyze videos from the official AWS playlist on introductory Amazon SageMaker videos, also available on YouTube. The notebook walks through transcribing and viewing Amazon A2I tasks for a video about Amazon SageMaker Jupyter Notebook instances. In Steps 3 and 4, we analyze results for a larger dataset of four videos. The following table outlines the videos that are used in the notebook, and how they are used.
Video # | Video Title | File Name | Function |
1 |
Fully-Managed Notebook Instances with Amazon SageMaker – a Deep Dive | Fully-Managed Notebook Instances with Amazon SageMaker – a Deep Dive.mp4 | Perform the initial transcription and viewing sample Amazon A2I jobs in Steps 1 and 2.Build a custom vocabulary in Step 3 |
2 |
Built-in Machine Learning Algorithms with Amazon SageMaker – a Deep Dive | Built-in Machine Learning Algorithms with Amazon SageMaker – a Deep Dive.mp4 | Test transcription with the custom vocabulary in Step 4 |
3 |
Bring Your Own Custom ML Models with Amazon SageMaker | Bring Your Own Custom ML Models with Amazon SageMaker.mp4 | Build a custom vocabulary in Step 3 |
4 |
Train Your ML Models Accurately with Amazon SageMaker | Train Your ML Models Accurately with Amazon SageMaker.mp4 | Test transcription with the custom vocabulary in Step 4 |
In Step 4, we refer to videos 1 and 3 as the in-sample videos, meaning the videos used to build the custom vocabulary. Videos 2 and 4 are the out-sample videos, meaning videos that our workflow hasn’t seen before and are used to test how well our methodology can generalize to (identify technical terms from) new videos.
Feel free to experiment with additional videos downloaded by the notebook, or your own content.
Step 1: Performing the initial transcription
Our first step is to look at the performance of Amazon Transcribe without custom vocabulary or other modifications and establish a baseline of accuracy metrics.
Use the transcribe
function to start a transcription job. You use vocab_name
parameter later to specify custom vocabularies, and it’s currently defaulted to None
. See the following code:
Wait until the transcription job displays COMPLETED
. A transcription job for a 10–15-minute video typically takes up to 5 minutes.
When the transcription job is complete, the results is stored in an output JSON file called YOUR_JOB_NAME
.json in your specified BUCKET
. Use the get_transcript_text_and_timestamps
function to parse this output and return several useful data structures. After calling this, all_sentences_and_times
has, for each transcribed video, a list of objects containing sentences with their start time, end time, and confidence score. To save those to a text file for use later, enter the following code:
To look at the distribution of confidence scores, enter the following code:
The following graph illustrates the distribution of confidence scores.
Next, we filter out the high confidence scores to take a closer look at the lower ones.
You can experiment with different thresholds to see how many words fall below that threshold. For this use case, we use a threshold of 0.4, which corresponds to 16 words below this threshold. Sequences of words with a term under this threshold are sent to human review.
As you experiment with different thresholds and observe the number of tasks it creates in the Amazon A2I workflow, you can see a tradeoff between the number of mis-transcriptions you want to catch and the amount of time and resources you’re willing to devote to corrections. In other words, using a higher threshold captures a greater percentage of mis-transcriptions, but it also increases the number of false positives—low-confidence transcriptions that don’t actually contain any important technical term mis-transcriptions. The good news is that you can use this workflow to quickly experiment with as many different threshold values as you’d like before sending it to your workforce for human review. See the following code:
You get the following output:
The following graph shows the distribution of confidence scores less than 0.4.
As you experiment with different thresholds, you can see a number of words classified with low confidence. As we see later, terms that are specific to highly technical domains are more difficult to automatically transcribe in general, so it’s important that we capture these terms and incorporate them into our custom vocabulary.
Step 2: Creating human review workflows with Amazon A2I
Our next step is to create a human review workflow (or flow definition) that sends low confidence scores to human reviewers and retrieves the corrected transcription they provide. The accompanying Jupyter notebook contains instructions for the following steps:
- Create a workforce of human workers to review predictions. For this use case, creating a private workforce enables you to send Amazon A2I human review tasks to yourself so you can preview the worker UI.
- Create a work task template that is displayed to workers for every task. The template is rendered with input data you provide, instructions to workers, and interactive tools to help workers complete your tasks.
- Create a human review workflow, also called a flow definition. You use the flow definition to configure details about your human workforce and the human tasks they are assigned.
- Create a human loop to start the human review workflow, sending data for human review as needed. In this example, you use a custom task type and start human loop tasks using the Amazon A2I Runtime API. Each time
StartHumanLoop
is called, a task is sent to human reviewers.
In the notebook, you create a human review workflow using the AWS Python SDK (Boto3) function create_flow_definition. You can also create human review workflows on the Amazon SageMaker console.
Setting up the worker task UI
Amazon A2I uses Liquid, an open-source template language that you can use to insert data dynamically into HTML files.
In this use case, we want each task to enable a human reviewer to watch a section of the video where low confidence words appear and transcribe the speech they hear. The HTML template consists of three main parts:
- A video player with a replay button that only allows the reviewer to play the specific subsection
- A form for the reviewer to type and submit what they hear
- Logic written in JavaScript to give the replay button its intended functionality
The following code is the template you use:
The {{ task.input.filePath | grant_read_access }}
field allows you to grant access to and display a video to workers using a path to the video’s location in an S3 bucket. To prevent the reviewer from navigating to irrelevant sections of the video, the controls
parameter is omitted from the video tag and a single replay button is included to control which section can be replayed.
Under the video player, the <crowd-text-area>
HTML tag creates a submission form that your reviewer uses to type and submit.
At the end of the HTML snippet, the section enclosed by the <script>
tag contains the JavaScript logic for the replay button. The {{ task.input.start_time }}
and {{ task.input.end_time }}
fields allow you to inject the start and end times of the video subsection you want transcribed for the current task.
You create a worker task template using the AWS Python SDK (Boto3) function create_human_task_ui. You can also create a human task template on the Amazon SageMaker console.
Creating human loops
After setting up the flow definition, we’re ready to use Amazon Transcribe and initiate human loops. While iterating through the list of transcribed words and their confidence scores, we create a human loop whenever the confidence score is below some threshold, CONFIDENCE_SCORE_THRESHOLD
. A human loop is just a human review task that allows workers to review the clips of the video that Amazon Transcribe had difficulty with.
An important thing to consider is how we deal with a low-confidence word that is part of a phrase that was also mis-transcribed. To handle these cases, you use a function that gets the sequence of words centered about a given index, and the sequence’s starting and ending timestamps. See the following code:
For every word we encounter with low confidence, we send its associated sequence of neighboring words for human review. See the following code:
For the first video, you should see output that looks like the following code:
As you’re completing tasks, you should see these mis-transcriptions with the associated video clips. See the following screenshot.
Human loop statuses that are complete display Completed
. It’s not required to complete all human review tasks before continuing. Having 3–5 finished tasks is typically sufficient to see how technical terms can be extracted from the results. See the following code:
When all tasks are complete, Amazon A2I stores results in your S3 bucket and sends an Amazon CloudWatch event (you can check for these on your AWS Management Console). Your results should be available in the S3 bucket OUTPUT_PATH
when all work is complete. You can print the results with the following code:
Step 3: Improving transcription using custom vocabulary
You can use the corrected transcriptions from our human reviewers to parse the results to identify the domain-specific terms you want to add to a custom vocabulary. To get a list of all human-reviewed words, enter the following code:
We want to parse through these words and look for uncommon English words. An easy way to do this is to use a large English corpus and verify if our human-reviewed words exist in this corpus. In this use case, we use an English-language corpus from Natural Language Toolkit (NLTK), a suite of open-source, community-driven libraries for natural language processing research. See the following code:
The words you find may vary depending on which videos you’ve transcribed and what threshold you’ve used. The following code is an example of output from the Amazon A2I results of the first and third videos from the playlist (see the Getting Started section earlier):
With these technical terms, you can now more easily manually create a custom vocabulary of those terms that we want Amazon Transcribe to recognize. You can use a custom vocabulary table to tell Amazon Transcribe how each technical term is pronounced and how it should be displayed. For more information on custom vocabulary tables, see Create a Custom Vocabulary Using a Table.
While you process additional videos on the same topic, you can keep updating this list, and the number of new technical terms you have to add will likely decrease each time you get a new video.
We built a custom vocabulary (see the following code) using parsed Amazon A2I results from the first and third videos with a 0.5 THRESHOLD
confidence value. You can use this vocabulary for the rest of the notebook:
After saving your custom vocabulary table to a text file and uploading it to an S3 bucket, create your custom vocabulary with a specified name so Amazon Transcribe can use it:
Wait until the VocabularyState
displays READY
before continuing. This typically takes up to a few minutes. See the following code:
Step 4: Improving transcription using custom vocabulary
After you create your custom vocabulary, you can call your transcribe function to start another transcription job, this time with your custom vocabulary. See the following code:
Wait for the status of your transcription job to display COMPLETED
again.
Write the new transcripts to new .txt files with the following code:
# Save the improved transcripts
i = 1
for list_ in all_sentences_and_times_custom: file = open(f"improved_transcript_{i}.txt","w") for tup in list_: file.write(tup['sentence'] + "n") file.close() i = i + 1
Results and analysis
Up to this point, you may have completed this use case with a single video. The remainder of this post refers to the four videos that we used to analyze the results of this workflow. For more information, see the Getting Started section at the beginning of this post.
To analyze metrics on a larger sample size for this workflow, we generated a ground truth transcript in advance, a transcription before the custom vocabulary, and a transcription after the custom vocabulary for each video in the playlist.
The first and third videos are the in-sample videos used to build the custom vocabulary you saw earlier. The second and fourth videos are used as out-sample videos to test Amazon Transcribe again after building the custom vocabulary. Run the associated code blocks to download these transcripts.
Comparing word error rates
The most common metric for speech recognition accuracy is called word error rate (WER), which is defined to be WER =(S+D+I)/N, where S, D, and I are the number of substitution, deletion, and insertion operations, respectively, needed to get from the outputted transcript to the ground truth, and N is the total number of words. This can be broadly interpreted to be the proportion of transcription errors relative to the number of words that were actually said.
We use a lightweight open-source Python library called JiWER for calculating WER between transcripts. See the following code:
For more information, see JiWER: Similarity measures for automatic speech recognition evaluation.
We calculate our metrics for the in-sample videos (the videos that were used to build the custom vocabulary). Using the code from the notebook, the following code is the output:
To calculate our metrics for the out-sample videos (the videos that Amazon Transcribe hasn’t seen before), enter the following code:
Reviewing the results
The following table summarizes the changes in WER scores.
If we consider absolute WER scores, the initial WER of 5.18%, for instance, might be sufficiently low for some use cases—that’s only around 1 in 20 words that are mis-transcribed! However, this rate can be insufficient for other purposes, because domain-specific terms are often the least common words spoken (relative to frequent words such as “to,” “and,” or “I”) but the most commonly mis-transcribed. For applications like search engine optimization (SEO) and video organization by topic, you may want to ensure that these technical terms are transcribed correctly. In this section, we look at how our custom vocabulary impacted the transcription rates of several important technical terms.
Metrics for specific technical terms
For this post, ground truth refers to the true transcript that was transcribed by hand, original transcript refers to the transcription before applying the custom vocabulary, and new transcript refers to the transcription after applying the custom vocabulary.
In-sample videos
The following table shows the transcription rates for video 1.
The following table shows the transcription rates for video 3.
Out-sample videos
The following table shows the transcription rates for video 2.
The following table shows the transcription rates for video 4.
Using custom vocabularies resulted in an 80-percentage point or more increase in the number of correctly transcribed technical terms. A majority of the time, using a custom vocabulary resulted in 100% accuracy in transcribing these domain-specific terms. It looks like using custom vocabularies was worth the effort after all!
Cleaning up
To avoid incurring unnecessary charges, delete resources when not in use, including your S3 bucket, human review workflow, transcription job, and Amazon SageMaker notebook instance. For instructions, see the following, respectively:
- How do I delete an S3 Bucket?
- Delete a Flow Definition
- DeleteTranscriptionJob
- Cleanup: SageMaker Resources
Conclusion
In this post, you saw how you can use Amazon A2I human review workflows and Amazon Transcribe custom vocabularies to improve automated video transcriptions. This walkthrough allows you to quickly identify domain-specific terms and use these terms to build a custom vocabulary so that future mentions of term are transcribed with greater accuracy, at scale. Transcribing key technical terms correctly may be important for SEO, enabling highly specific textual queries, and grouping large quantities of video or audio files by technical terms.
The full proof-of-concept Jupyter notebook can be found in the GitHub repo. For video presentations, sample Jupyter notebooks, and more information about use cases like document processing, content moderation, sentiment analysis, object detection, text translation, and more, see Amazon Augmented AI Resources.
About the Authors
Jasper Huang is a Technical Writer Intern at AWS and a student at the University of Pennsylvania pursuing a BS and MS in computer science. His interests include cloud computing, machine learning, and how these technologies can be leveraged to solve interesting and complex problems. Outside of work, you can find Jasper playing tennis, hiking, or reading about emerging trends.
Talia Chopra is a Technical Writer in AWS specializing in machine learning and artificial intelligence. She works with multiple teams in AWS to create technical documentation and tutorials for customers using Amazon SageMaker, MxNet, and AutoGluon. In her free time, she enjoys meditating, studying machine learning, and taking walks in nature.